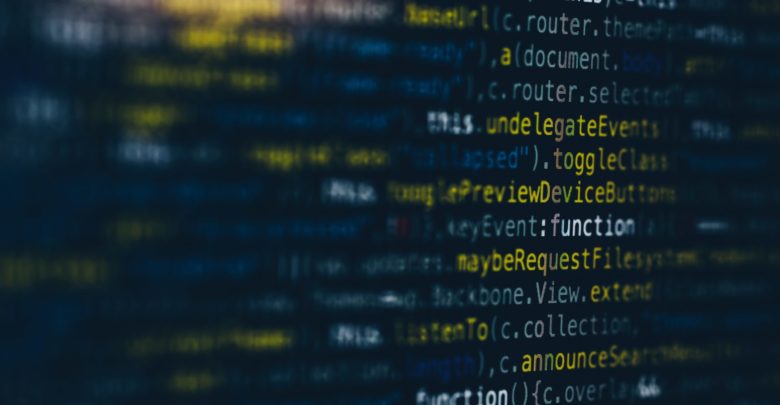
U of A researchers were involved in a study that found ways to reduce gender bias in natural language processing.
The study began at Lancaster University in England with the goal to make natural language processing fairer and reduce gender bias, specifically in the labour market. The study is part of a larger initiative, BIAS: Responsible AI for Gender and Ethnic Labour Market Equality, and comes as the newest leap forward in the development of natural language processing.
Lei Ding, a University of Alberta graduate student in the department of mathematical and statistical sciences, is the computational team leader of the project. Ding explained natural language processing as a computer’s way of understanding language.
“A computer is just like a calculator, you can only calculate numbers … if you need the machine to understand text, you need to first convert these things into numbers.”
A simple example of the use of natural language processing is when email inboxes automatically classify whether a text is spam or not.
A primary application of this specific artificial intelligence (AI) would be job advertisements. The AI would make the wording in the job posting more equitable. Ding added that AI itself is not inherently prejudiced, as programmers include their unconscious bias in their code.
“If you’re reading a job post written in very masculine words, maybe a female … will not apply for [the] job,” Ding said. “What we have done is to … try to nullify those very sensitive words, and then make the whole job ad to be more gender neutral.”
The study is a multidisciplinary project since AI is not only “related to machine learning, to statistics, [but] also to sociology.” The study has contributing researchers ranging from computer scientists, statisticians, and sociologists.
The goal of the project is for the algorithm to rewrite job text to make it more equitable for those who apply. Ding and his team have been tackling some complications of the project, one of them being evaluation.
“[Evaluation] is pretty tricky because there are not many golden standards for what is biased or not.”
A concern with this new technology is that its efficacy may scrub away too much information from a given word or sentence. Ding gave the example of the word: nurse, in which old models of natural language processing would associate the word with being more feminine.
“[With nurse], we want to remove the gender information related to females, but we don’t want to remove other relevant information, such as hospital or doctor.”
To address this problem, the multi-disciplinary team combines word lists generated by sociologists with programmers who use advanced machine learning to expand that list, with synonyms and similar words.
Ding and his team at the U of A continue their work and the advancement of their study, as well as the way AI is perceived in our modern world.
“[In the future] we want to get a better way to evaluate the bias … we want the whole context of the job posts to be more amiable other than looking at some keywords.”