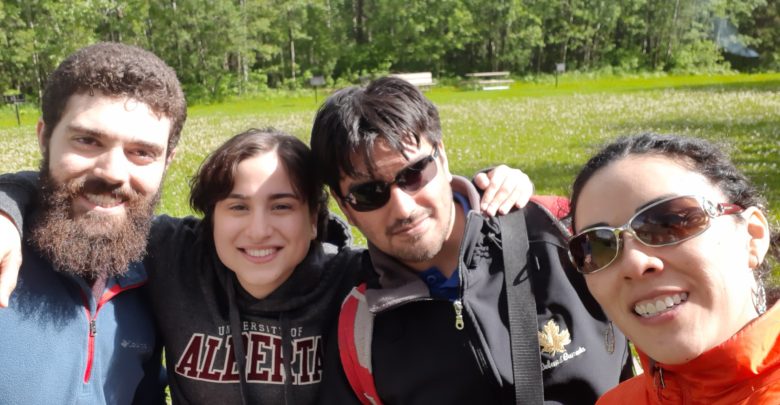
Researchers at the University of Alberta are carrying on the legacy of a coworker they lost in the Flight PS 752 crash.
Pouneh Gorji was a graduate student completing a master of science in computing science when she became one of the 10 U of A community members to pass away on Flight PS 752. She has posthumously been made the second author of a paper describing a deep learning model she helped to develop, which can more accurately diagnose hip dysplasia, glaucoma, and fatty liver, in patients. Deep learning refers to machine learning based on artificial neural networks.
Roberto Vega, the lead author of the paper and researcher in the department of computing science, said he considered Gorji his best friend and worked closely with her to bring this research to fruition.
“It took us one and a half years and three rejections to get this paper done, so we [were] very satisfied when we finally got it right,” Vega said. “Unfortunately, [Pouneh] couldn’t see that, but still, her work was a crucial part for getting acceptance [to publish our findings].”
Vega said he was “glad” that the researchers could honour Pouneh’s contributions by having her name appear in a paper.
“She’s the second author not because of charity, not because of what happened to her, it is because she earned that,” he said.
Gorji was an active member of the computing science department’s Graduate Student Association. She was set to finish her masters studies in the summer of 2020, and has since been recognized with a posthumous degree.
Gorji was appointed “Vice-President of Fun” during time at lab, Vega said
Vega said Gorji’s supervisor, Russ Greiner, appointed her “Vice-President of Fun.” Gorji organized movie nights, bowling games, and trips to Elk Island. She and her husband, Arash Pourzarabi — a U of A researcher in reinforcement learning — passed away in Flight PS 752 just days after they were married.
“I miss her a lot and I still think about her basically every day,” Vega said. “[Her loss] was felt in the entire department. We were always in each other’s office, and my wife and I had a nice connection with both her and her husband. It was my wife who organized her bachelorette party before she was married.”
According to Vega, Gorji’s most significant contribution to the project was developing a solution to the problem of diagnosing fatty liver.
“I didn’t even know that I had a problem with my approach [for diagnosing fatty liver],” said Vega. “It was only when [Gorji] started working on this that [I realized] ‘Oh, this is a flaw, this is a very big problem [and] it’s an important one.’”
Vega explained that to diagnose fatty liver, physicians look at whether the liver or a surrounding vein or artery appears “brighter” in the scan compared to other organs, and whether the contours of the liver are defined. These features can be highly subjective, so the probabilistic model that worked for hip dysplasia and glaucoma — other diseases their project worked to diagnose — wouldn’t work in this case.
Together with Gorji, Vega said they created a mathematical framework that combined what he called “the traditional method” of allowing the AI to learn on its own based on a sample of images, with the probabilistic model where the AI was given specific, quantifiable patterns to detect.
“Pouneh and I were working on [it] for several months until we got an answer that was successful, so her contribution was vital,” Vega said. “She was very, very smart and I enjoyed working with her a lot. She was a hard worker.”
Deep learning follows human physicians’ practices to diagnose
Slightly different from the process of diagnosing fatty liver disease, the researcher’s AI acquires how human physicians diagnose hip dysplasia and glaucoma by measuring the distance and angle between bones and the diameter in some parts of the eye respectively.
Based on these quantifiable components and the physician’s diagnosis associated with the scans, the AI is able to return the probability that the scan reveals a disease or not.
“We kind of ‘force’ AI or machine learning to adhere to a set of clinical practices that the physicians need to,” Vega explained.
He said this restrained version of AI guards against some typical pitfalls, such as the AI being skewed based on a small or poorly representative training sample size. Additionally, while this model is in itself a black box — meaning not readily explainable to humans — Vega shared some ideas for how this newly published research might be applied in a clinical setting.
“One way of giving the physician confidence is to use the [AI] system to give you an intermediate step,” he said.
One example he gave would be to use the same AI to highlight the parts of the image it believes show affectation of a disease, instead of giving the diagnosis directly, so the model is more of a tool for physicians rather than a replacement.
Vega said his background in computational psychiatry was inspirational in this research. It was when he discovered that mental illnesses could not be diagnosed by an AI or psychiatrist based on patient scans alone that he decided to incorporate the medical knowledge of professionals in the field into the deep learning system.
“I found I could apply the same set of techniques that we tried to use in computational psychiatry to these other problems in radiology,” he said.
He explained that in computational psychiatry, a similar tool for deep learning is used to advance despite the AI having few brain scans to learn from.
“We tried to use the same [technology] in radiology,” Vega said.
Vega, who has been working in the computing science for health care area since he obtained a bachelor’s degree in Mexico, said he was initially interested in studying AI because he believed that machine learning could help physicians deliver better health care.
“I believe that machine learning has the potential to improve the way in which we deliver health care now, and not only in countries like in Canada, but also in developing countries where the medical system is not as efficient,” Vega said.